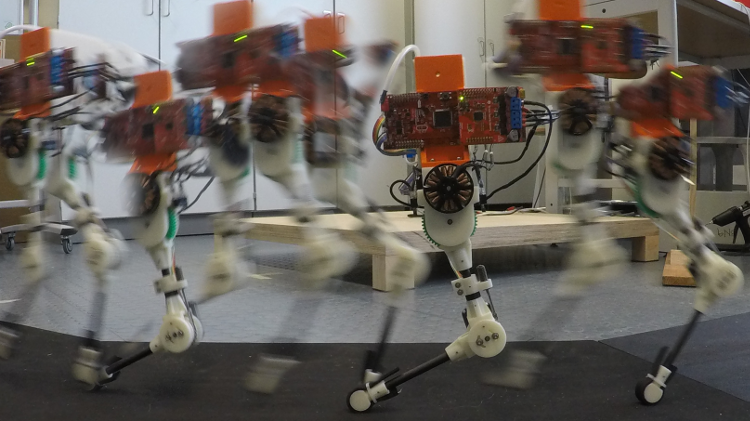
Learning to hop on a single legged robot
Locomotion in animals is potentially the result of clever coupling between morphology and neural control. Visibly, this results in agile, efficient and graceful animal movement. In engineering, this coupling is often mimicked in robot design. For example, to allow compliant control of an end-effector, reflected inertia is minimized by placing the actuators close to the body and minimizing gear ratio. Many robots are specifically built around the concept of passive dynamic walkers, to exploit passive stability properties and make the overall system easier to control.
This concept, to design mechanics that allow effective control and conversely, to design control policies that effectively take advantage of the mechanical design, is loosely covered under the term of exploiting natural dynamics.
We are investigating how to exploit natural dynamics in the specific context of learning: how might a certain morphology influence the task of learning motor control? Can we use these ideas to build robots that are more amenable to machine learning?